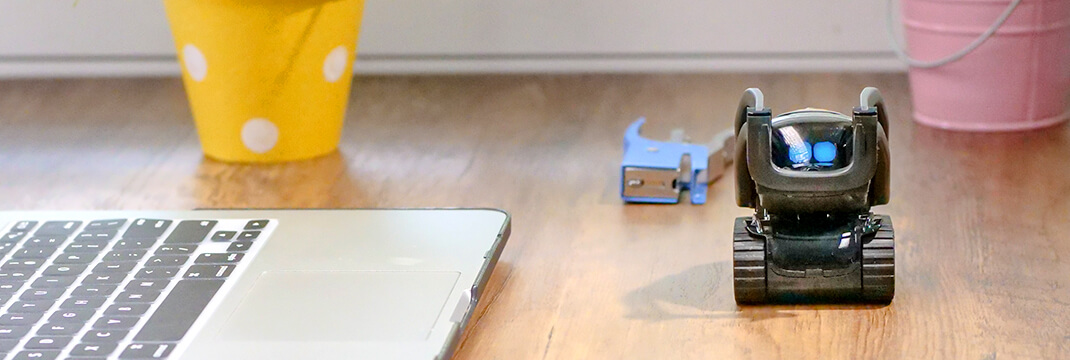
3.0 Research Methodology
3.1 Introduction
This chapter will examine the methodology used to carry out this research study. The quantitative method of research will be in use for this study. This will help to obtain empirical data on the type of innovation adopted by various firms. This quantitative assessment will go a long way to give the type of innovation used by most firms, whether it is open or closed innovation. This chapter will give an in-depth analysis of the research design employed for the primary data along with its expected challenges, the method that will be used in data collection, and the statistical methods of data analysis that will be employed to obtain results in line with the expected outcome (Lucas 1999). Among the statistical methods employed, there are descriptive statistics, which will help to present information from the data collected in a convenient and understandable way. Stepwise multiple regressions will help in analyzing the relationship between a criterion variable and several predictor variables.
3.2 Research Design
To obtain data about the method of innovation used by various firms, questionnaires were polled to the sampled firms in Taiwan as identified using the sampling frame. The method used was a telephone interview. The interviewer called the firms and asked the questions contained in the questionnaires.
3.3 Primary Research
The questionnaires administered through telephone interviews with the respondents about their methods of innovation, and were broken down to several related questions. The questions were short and straightforward to avoid wasting the time of the respondents. Also, these questions were supposed to be answered by the respondents to answer easily without straining. The data was from respondents sampled from a variety of firms at the same point in time and, hence, was cross sectional data. Primary research was opposed to secondary research because the targeted issues are addressed, and the research moves in the direction to favor of the researchers. Data interpretation is better since the researcher controls the variables. There is efficient spending of resources since the research focuses on a specific issue unlike secondary research whereby the researcher has to get variables to fit the situation. Secondary data may not also be recent and, therefore, might not show the current situation correctly. A propriety issue is another advantage since most researchers can carry out research and keep the findings to themselves because they own it. This might lead to competitors using secondary research being disadvantaged. Primary research also has some disadvantages, these are: high cost of carrying out research, time consuming since the researcher has to get responses from the respondents no matter how large the sample is, sometimes inaccurate feedbacks can be obtained, and a higher amount of resources are required for the exercise.
Along with the first order offer - 15% discount (code firstpaper15), you save an extra 10% since we provide 300 words/page instead of 275 words/page.
3.4 Primary Data
An advantage of using primary data is that researchers are collecting information for specified purposes. In essence, the questions the researchers ask are framed to elicit the data that will help them with their study. Researchers collect the data themselves, using surveys, interviews, and direct observations. This study will seek to get answers concerning the effects of applying technologically driven methods of production to the performance of the firms. The firms will be interviewed over the telephone. They will be asked questions about how they have been carrying on with their production activities and their interaction with other firms in terms of innovation.
The answers of the firms’ representatives will be considered primary data. From these answers, the researcher will analyze and get the exact contribution of technology to the productivity of a firm.
3.5 Sample and Data Collection
A sample of 100 firms was from a sampling frame of 451 firms from the Taiwan medical and biotechnology industry association in Taiwan. The inclusion of any firm in the sample was purely random. The phone numbers of each of the sampled firms were taken from the directory. Then, the owners of these phone numbers were called and interviewed. Respondents who were busy at the time of the interviews rescheduled for other times. These callbacks were done efficiently.
You may find this article Pay for Essay Help
useful.
3.6 Variables
3.6.1 Independent Variables: Open or Closed Innovation
Independent variables used in this study based on whether the firm used closed or open innovation. All the questions required the firms to give quantified amount of a certain variable related to the open or closed innovation method. The firms were required to:
Give the number of projects per year they have done in alliance with universities, which are centers of innovation. The relationship that exist between firms or collaboration that exist between the firms with the aim of innovation was quantified by the number of projects they managed to realize within the year. They were also to give an account of whether they had bought or sold patents, as well as the exact number. The total expenditure of technology acquisition through licensing contracts was also to be given if they had contracted R and D. Finally, they were to give the expenditure they had incurred in R and D.
3.6.2 Dependent Variables: Performance
The criterion variables based on the performance from the acquired innovation either open or closed. The performance of the firms was measured in terms of the increased turnover to the affected firm(s). The firms were required to submit the following information:
The firm’s turnover that was attributable to the improvement in technology or new products, the number of the new applied patents, and the number of the new launched products.
3.7 Questionnaire Design
The questionnaire consists of three sections: section 1, section 2, and section 3, which focus on the following areas:
- Section 1: It deals with the firms individual characteristics, such as its location, number of years of operation, and distances from similar firms, research institutes, and trained labor.
- Section 2: It deals with questions related to the type of innovation adopted by the firms whether open or closed.
- Section 3: It aims at assessing the performance of the firms because of interacting with other firms or adopting innovative methods of production or operations.
3.8 Advantages and Disadvantages of Phone Interviews
The reason why telephone interview thought as the most efficient is that telephone interviews can be easily arranged and are less time consuming than face-to-face interviews. The cost of interviewing a large number of respondents is less as compared to face-to-face interviews. The list of questions in the questionnaire is standardized, and therefore, one expects a straightforward answer. However, this method also poses some challenges. For instance, some of the respondents may not be familiar with the format or are uncomfortable in using the gadget; this may spark irrelevant answering of the questions. It is also hard to study the mood and body language of the respondents, which is essential in a survey. Another problem with phone interviews is that some respondents may give answers based on what the researcher want to hear rather than the truth; this is for fear that that information may be used against them.
3.9 Data Analysis Technique
Data was entered and analyzed using STATA 10 for windows (StataCorp LP, College Station, TX, USA) software. The variables were coded in case of ordinal and nominal variables before interviewing the respondents. Data were cleaned after the entry in order to deal with non-response ensuring that the variables of interest were covered. Then testing of the data was carried out including descriptive.
3.10 Data Analysis
In this section, the statistical analysis of the data was carried out in order to produce the desired results.
3.10.1 Descriptive Statistics
The major advantage of descriptive statistics is to present data in a convenient and understandable form. In the data, the measures of central tendencies (mean, median, and mode) and also measures of dispersion (Standard deviation and variance) are useful since most of the variables are measured in the scale ratio for the categorical variables, they are presented as percentages. Since there is a cross sectional data, the histogram should be looked at (Baltagi 2007). The histogram, due to its defined shape, offers a clue about any issues affecting the data. Differences in the characteristics of continuous variables were tested using ANOVA, and for the categorical variables, chi squared tests were used.
Invite your friends and get bonus from each order they
have made!
3.10.2 Pareto Analysis
The Pareto chart looks like the bar chart, but the bars are arranged in a specified way. It will show the effect of different levels of innovation to the performance of a firm in a descending order of magnitude. It is able to single out the most important innovation means that will adversely increase the performance of a firm in terms of its productivity (Baltagi 2007). For this case Pareto effect might be strong that its significance might be obvious, but C-charts will also be important in order to check the assumption of poisson distribution.
3.10.3 Correlation
Correlation determines whether a relationship exists between two variables, as well as its direction and magnitude. The Pearsons product moment correlation coefficient (r), two sets of measurements are obtained from one particular individual or from a pair of individuals with a common basis. The values of r vary between +1 and -1 where by both of these extremes show perfect relationship between variables whereas 0 indicate no relationship. In this very case, we shall use correlation coefficient to test the strength of the predictions to determine the variables that will go in first in the multiple regression equation.
3.10.4 Testing the Assumptions of Regression analysis
Regression analysis is based on correlation; hence, the linearity concept between the dependent and the independent variables is crucial. To test this, we will use residual plots.
Homoskedasticity is the assumption that two variables have a common variance. Residual plots and the Levene test for homogeneity of the variance will test violation of this assumption (Cohen 2003). In this very case, they will be used, as well.
Independence of the error terms: in any regression, we assume that the predicted value is in no way related to any other prediction. We will have residual plots for this and also the Durbin-Watson statistic which will test for any serial correlation of simultaneous error terms and if it is significant, it will show that they are independent (Hausman, 1978).
Lastly, we test normality of the error distribution. We assume that differences between the obtained and predicted dependent variable values are normally distributed. We will construct a histogram of residuals to give a picture of whether their distribution is approximately normal.
3.10.5 Statistical (Stepwise) Regression
Regression involves the relationship between variables. The size of the sample impacts on the statistical power of the significance testing in multiple regression. Power as applied to multiple regression criteria refers to the probability of detecting as significant a specified value of the coefficient of determination, R2 at a specified level of significance (Hair et al. 2006). This implies that for a specific level of significance and the number of independent variables, There is a certain sample size that produces a significant R2 (Kumar 1999).
For this particular regression model, the order of entry of the explanatory variables into the model based on a specified statistical criterion; therefore, the variables that have a higher correlation with the dependent variable were afforded priority to the model without any theoretical considerations. Firstly, the independent variables (alliance with the university, inter-firm corporation, expenditure of technology acquisition, firms contracted R & D expenditure, and expenditure of internal R & D) will be entered into the models simultaneously. That is the forced entry criteria to find out whether each of the variables was significant even after the non-useful covariates were excluded (Hausman 1978).
The nested regression models were used in order to investigate whether firms performance was directly associated with open and closed innovation with the performance being in terms of firms turnover attributed to technology, the number of new and applied patents, and the number of new launched products.
3.10.5.1 Evaluating the strength of the prediction equation
A measure of the strength of the prediction equation that is developed is R square (coefficient of determination). In the regression model, R square is the square of the correlation coefficient between the observed value of the dependent variable and the predicted value obtained from the fitted regression line. If all observations fall on the regression line, R square is 1, and we assume a perfect relationship between the dependent and the independent variables. An R square of 0 indicates that no linear relationship between the predictor and the response variable. Therefore, the distance between 0 and 1 of R Square gives an increasing magnitude of the strength of the relationship. To test the hypothesis that no linear relationship exists between the predictor and the response variables, the F-Value in the ANOVA is used. If the probability associated with the F-Statistic is small, the hypothesis that R-Square= 0 is rejected (Cohen 2003).
3.10.5.2 Multicollinearity
This refers to the situation whereby, the predictor variables are highly correlated amongst themselves (Lucas 1999). This implies that there is sharing of the predictive power among the predictor variables. Therefore, it is difficult to assess the contribution of each of the predictor variables. To ensure this is not the case, we compute the Tolerance and Variance Inflation factor (VIF). Tolerance is calculated by 1-R2 where R2 is the coefficient of determination. This clearly shows that the tolerance value is the proportion of variance that cannot be accounted for by other predictors. Hence, a very small value of tolerance is interpreted to mean that there is sharing of the predictive power between the predictor variables (Cohen 2003). VIF value is computed as the reciprocal of the tolerance value; predictor variables with VIF less than 10 require further investigation.
4.0 Findings
4.1 Introduction
This chapter is devoted to the analysis of the results obtained from the 100 firms sampled. It will be divided into sections of descriptive statistics, Pareto analysis, Pearson’s product moment correlation, Testing the assumptions of regression analysis, and finally, statistical regression will be preformed.
4.2 Descriptive Statistics
The Statistics table above presents the mean, std deviation minimum value, and maximum value. The results show that the 100 respondents in the survey have a mean of 16.14 projects that they have done with related firms, and a standard deviation of 16.61. The projects done in cooperation with other firms had a mean of 15.68 and a standard deviation of 14.58. The turnover of the firms that is attributed to technology had a mean of $55114.88 with a standard deviation of 88008.65. New patents and new products had a mean of 14.45 and 14.63 respectively. The expenditure on technology, expenditure on contracted R & D, and expenditure on internal R & D variables were nominal, and therefore, their mean and standard deviation are not meaningful.
4.3 Pareto Charts
This Pareto chart shows that the turnover of 54872 was the highest attributed interrelations between firms with a percentage of 15 %.
This Pareto chart shows that the turnover of 101319 was the highest attributed interrelations between firms with a percentage of 22 %.
4.4 Correlation
The correlation between the association of the university and the turnover is highly positively related and statistically significant (r=0.0881). The correlation between the turnover and the firm and is positive, but weak (r=0.2415). From the table above, it is evident that the correlation between buying and selling of patents is a bit weak, but positive with a value of r=0.4593. The correlation between the alliance with the university is positive with r=0.39
Histogram
The histogram for the Turnover attributed to the firms’ technology shows that it is skewed towards one direction, meaning that still few firms embrace technology.
Scatter Plots
As it can be seen from the scatter plot, there is a linear relationship between the variables of the Turnover and the alliance with the university. This implies that an increase in the alliance between the firms and the universities implies an increase in the turnover of the firms.
As it is evident from the scatter plot, there is a linear relationship between the variables of the Turnover and the interrelationship between firms, which are the major variables in the following study.
4.5 Statistical Regression:
Solely, the method of the entry of the variables will follow a predefined statistical criterion. Variables that correlate more strongly with the dependent variable will be given a higher priority in the entry.
4.5.1 Model 1
The independent variables will be an alliance between universities and firms, interrelationships among firms, as well as buying and selling of patents while the dependent variable will be turnover attributed to innovation.
Model | R | R Square | Adjusted R Square | Std. Error of the Estimate | Change Statistics | Durbin-Watson | ||||
R Square Change | F Change | df1 | df2 | Sig. F Change | R Square Change | F Change | df1 | df2 | Sig. F Change | |
1 | .459(a) | .211 | .203 | 78574.397 | .211 | 26.201 | 1 | 98 | .000 | |
2 | .496(b) | .246 | .230 | 77226.431 | .035 | 4.451 | 1 | 97 | .000 | 1.506 |
ANOVA(c)
Model | Sum of Squares | df | Mean Square | F | Sig. | |
1 | Regression | 161761067047.762 | 1 | 161761067047.762 | 26.201 | .000(a) |
Residual | 605045710360.798 | 98 | 6173935820.008 | |||
Total | 766806777408.560 | 99 | ||||
2 | Regression | 188306377036.263 | 2 | 94153188518.132 | 15.787 | .000(b) |
Residual | 578500400372.297 | 97 | 5963921653.323 | |||
Total | 766806777408.560 | 99 |
a Predictors: (Constant), Buying/Selling of Patents
b Predictors: (Constant), Buying/Selling of Patents, Interrelationship between firms
Coefficients(a)
Model | Unstandardized Coefficients | Standardized Coefficients | t | Sig. | 95% Confidence Interval for B | Collinearity Statistics | ||||
B | Std. Error | Beta | Lower Bound | Upper Bound | Tolerance | VIF | B | Std. Error | ||
1 | (Constant) | 17071.214 | 10815.695 | 1.578 | .118 | -4392.180 | 38534.608 | |||
Buying/Selling of Patents | 3375.658 | 659.481 | .459 | 5.119 | .000 | 2066.939 | 4684.377 | 1.000 | 1.000 | |
2 | (Constant) | 1248.882 | 13009.428 | .096 | .924 | -24571.230 | 27068.995 | |||
Buying/Selling of Patents | 3204.908 | 653.201 | .436 | 4.906 | .000 | 1908.485 | 4501.332 | .985 | 1.016 | |
Interrelationship between firms | 1131.804 | 536.467 | .188 | 2.110 | .037 | 67.065 | 2196.543 | .985 | 1.016 |
Excluded Variables(c)
Model | Beta In | t | Sig. | Partial Correlation | Collinearity Statistics | |||
Tolerance | VIF | Minimum Tolerance | Tolerance | VIF | Minimum Tolerance | Tolerance | ||
1 | Alliance with Universities | -.038(a) | -.409 | .683 | -.042 | .928 | 1.078 | .928 |
Interrelationship between firms | .188(a) | 2.110 | .037 | .209 | .985 | 1.016 | .985 | |
2 | Alliance with Universities | -.127(b) | -1.297 | .198 | -.131 | .800 | 1.250 | .800 |
a Predictors in the Model: (Constant), Buying/Selling of Patents
b Predictors in the Model: (Constant), Buying/Selling of Patents, Interrelationship between firms
c Dependent Variable: Turnover Attributed to Technology
4.5.1.1 Prediction Equation
The relevant facts in the calculation of the predicted firms output are presented in the coefficients table. An examination of this table shows that only two of the 3-predictor variables were entered into the prediction equation (Yafee, 2001). This indicates that the alliance between firms and the universities is not a significant determinant of the turnover of a firm whereas buying and selling of patents and the interrelationship between firms are. To come up with the level of turnover attributed to the two predictors, we check the unstandardized coefficients.
Predicted Turnover Attribution= 1248.88 + (Buying of patents * 3409.2) +(Interrelation between firms * 1131.8)
4.5.1.2 Evaluating the Strength of the Prediction Equation
Testing the hypothesis of no linear relationship between the dependent and the independent variables, we check the ANOVA table. The F-Value in the ANOVA table serves to test how well the regression model fits the data (Yafee 2001). The computed F-statistic is 15.78, with an observed level of significance of less than 0.001. Therefore, we reject the hypothesis that there is no linear relationship between the predictor and dependent variables.
4.5.1.3 Identifying Multicollinearity
A problem comes up if the predictor variables are correlated among themselves, this is caused by sharing of prediction power. In this case, both the tolerance value (greater than 0.1) and the VIF value (less than 10) are acceptable, and hence, multicollinearity is not a problem for this model.
4.5.1.3 Identifying independent Relationships
Having established that multicollinearity has no effect on the model, we can assess the relative contribution of each of the predictor variables by controlling effects of the other predictor variable in the equation. In this case, we interpret the unstandardised regression coefficients (Beta). We obtain all the beta weights from the coefficients table above. The magnitude of the Beta weights has a direct impact on the direction and strength of the independent relationship (Yafee 2001). From the table, it is evident that buying and selling of patents has the strongest relationship with the Turnover of the firms as compared to interrelationships between firms. This implies that the more the firms bought or sold patents, the higher output they obtained. (Buying/ selling of patents: Beta=0.436, t=4.9, p<0.05). The positive coefficient associated with interrelationships between firms shows that the more the firms interrelated to invent, the more output from them is needed (Interrelationships among firms: Beta= 0.188, t=2.1, p<0.05).
4.5.2 Model 2
The independent variables will be alliance between universities and firms, interrelationships among firms, and buying and selling of patents while the dependent variable will be the number of new patents.
ANOVA(b)
Model | Sum of Squares | df | Mean Square | F | Sig. | |
1 | Regression | 4916.049 | 1 | 4916.049 | 30.147 | .000(a) |
Residual | 15980.701 | 98 | 163.068 | |||
Total | 20896.750 | 99 |
a Predictors: (Constant), Buying/Selling of Patents
b Dependent Variable: Number of new patents
Coefficients(a)
Model | Unstandardized Coefficients | Standardized Coefficients | t | Sig. | 95% Confidence Interval for B | Collinearity Statistics | ||||
B | Std. Error | Beta | Lower Bound | Upper Bound | Tolerance | VIF | B | Std. Error | ||
1 | (Constant) | 7.818 | 1.758 | 4.448 | .000 | 4.330 | 11.306 | |||
Buying/Selling of Patents | .588 | .107 | .485 | 5.491 | .000 | .376 | .801 | 1.000 | 1.000 |
a Dependent Variable: Number of new patents
Collinearity Diagnostics(a)
Model | Dimension | Eigenvalue | Condition Index | Variance Proportions | |
(Constant) | Buying/Selling of Patents | (Constant) | Buying/Selling of Patents | ||
1 | 1 | 1.687 | 1.000 | .16 | .16 |
2 | .313 | 2.322 | .84 | .84 |
a Dependent Variable: Number of new patents
4.5.2.1 Prediction Equation
The information that is relevant to the predicted acquiring of new patents is found on the coefficients table. A close examination of this table shows that only one of the 3- predictor variables is relevant to the acquiring of new patents. This shows that the alliance between firms and universities is not significant in the number of patents that firms acquire. The exact contribution of buying and selling of patents can be determined using the beta weights.
Predicted acquiring of new patents = 7.818 + (Buying and selling of patents *0.588)
4.5.2.2 Evaluation of the Strength of the predicted Equation
To test the hypothesis that there is no linear relationship between the predictor variable (Buying and Selling of patents) and the dependent variable (Acquiring of new patents), we review the ANOVA table. The F-value is an indicator of how well the model fits the data. In this case, the F-value is 30.147 with a p-value <0.001. Hence, the hypothesis that there is no linear relationship between the predictor and the dependent variable is rejected.
4.5.2.3 Identifying independent relationships
From the beta weights, it is evident that the buying and selling of patents have a beta weight of 0.485, a t value of 5.481 and a p-value less than 0.001. Hence, an increase in the buying and selling of new patents also increases the number of new patents.
4.5.3 Model 3
The independent variables will be alliance between universities and firms, interrelationships among firms, as well as buying and selling of patents while the dependent variable will be the number of new products.
ANOVA(c)
Model | Sum of Squares | df | Mean Square | F | Sig. | |
1 | Regression | 11002.467 | 1 | 11002.467 | 57.707 | .000(a) |
Residual | 18684.843 | 98 | 190.662 | |||
Total | 29687.310 | 99 | ||||
2 | Regression | 15550.199 | 2 | 7775.100 | 53.348 | .000(b) |
Residual | 14137.111 | 97 | 145.743 | |||
Total | 29687.310 | 99 |
a Predictors: (Constant), Alliance with Universities
b Predictors: (Constant), Alliance with Universities, Buying/Selling of Patents
c Dependent Variable: Number of new products
Excluded Variables(c)
Model | Beta In | t | Sig. | Partial Correlation | Collinearity Statistics | |||
Tolerance | VIF | Minimum Tolerance | Tolerance | VIF | Minimum Tolerance | Tolerance | ||
1 | Interelationship between firms | .002(a) | .023 | .982 | .002 | .850 | 1.177 | .850 |
Buying/Selling of Patents | .406(a) | 5.586 | .000 | .493 | .928 | 1.078 | .928 | |
2 | Interelationship between firms | -.007(b) | -.096 | .923 | -.010 | .849 | 1.177 | .800 |
a Predictors in the Model: (Constant), Alliance with Universities
b Predictors in the Model: (Constant), Alliance with Universities, Buying/Selling of Patents
c Dependent Variable: Number of new products
Collinearity Diagnostics(a)
Model | Dimension | Eigenvalue | Condition Index | Variance Proportions | ||
(Constant) | Alliance with Universities | Buying/Selling of Patents | (Constant) | Alliance with Universities | ||
1 | 1 | 1.699 | 1.000 | .15 | .15 | |
2 | .301 | 2.374 | .85 | .85 | ||
2 | 1 | 2.338 | 1.000 | .06 | .07 | .07 |
2 | .381 | 2.479 | .00 | .57 | .69 | |
3 | .282 | 2.880 | .94 | .36 | .24 |
a Dependent Variable: Number of new products
4.5.3.1 Prediction Equation
The relevant facts in the calculation of the predicted firms output are presented in the coefficients table. An examination of this table shows that only two of the 3-predictor variables were entered into the prediction equation. This indicates that buying and selling of patents is not a significant determinant of turnover of a firm whereas alliance with the university and the interrelationship between firms are. To come up with the level of the turnover attributed to the two predictors, we check the unstandardized coefficients.
Predicted Turnover Attribution= -0.393 + (University Alliance * .52) +(Interrelation between firms * .588)
4.5.3.2 Evaluating the Strength of the Prediction Equation
Testing the hypothesis of no linear relationship between the dependent and independent variables, we check the ANOVA table. The F-Value in the ANOVA table serves to test how well the regression model fits the data. The computed F-statistic is 53.348, with an observed level of significance of less than 0.001. Therefore, we reject the hypothesis that there is no linear relationship between the predictor and dependent variables.
4.5.3.3 Identifying Multicollinearity
A problem comes up if the predictor variables are correlated among themselves. This is caused by sharing of prediction power. In this case, both the tolerance value (greater than 0.1) and the VIF value (less than 10) are acceptable in this case, and hence, multicollinearity is not a problem for this model. Also, from the collinearity diagonistics table, the condition index summarizes the findings (Wackery 2009). Since the condition index is greater than 15, there is no multicollinearity problem.
4.5.3.3 Identifying independent Relationships
Having established that multicollinearity has no effect on the model, we can assess the relative contribution of each of the predictor variables by controlling effects of the other predictor variable in the equation (Wackery 2009). In this case, we interpret the unstandardised regression coefficients (Beta). We obtain all the beta weights from the coefficients table above. The magnitude of the Beta weights has a direct impact on the direction and strength of the independent relationship. From the table, it is evident that buying and selling of patents has the strongest relationship with the turnover of the firms as compared to interrelationships between firms. This implies that the more firms had alliances with universities, the higher output they obtained. (Buying /selling of patents: Beta=0.499, t=6.865, p<0.05). On the same note, the positive coefficient associated with interrelationships between firms shows that the more the firms interrelated to invent, the more the output from them (Interrelationships among firms: Beta= 0.406, t=5.586, p<0.05)
4.6 Conclusions
This chapter presented the statistical analysis and results obtained from the findings. Methods of describing the data were used; they included: Pareto graphs, scatter plots, and the histogram.
A correlation matrix was developed that compared the Pearson’s product moment correlations between different variables in the study.
The stepwise regression analysis was used to ascertain the relationship between the adoption of innovation by firms and the performance as depicted by the turnover of the firm. Acquiring of new technology and buying of new patents obtained from the statistical analysis, it can be concluded that the three independent variables have an effect on the performance of the firm but at different levels. The model was correctly specified as it is evident from the significance of the F- statistics and, therefore, can be used for inference purposes.
5.0 Discussion Recommendations and Conclusions
5.1 Overview of the Study
The aim of the study was to determine the effect of firms investing in innovation, either open or closed. Open innovation is defined by Chesbrough (2003, p. 36) as a situation whereby a company generates, develops and commercializes its own ideas. This approach calls for self-reliance: if you want something done right, you got to do it yourself. Internal R&D is strategic asset whereas closed innovation defined as a situation whereby a company commercializes both its own ideas, as well as innovations from other firms and seeks ways to bring its in-house ideas to market by deploying pathways outside its current business. The alliance with the universities for innovation purposes, interrelation between firms for purposes of innovation, and buying and selling of patents were the factors that directly implied innovation. In the study, we tried to establish the contribution of this innovation factors to the turnover of a firm, the tendency of the firm acquiring new patents and launching new products.
The research questions that were studied include:
- 1. Does the support of innovation by firms affect their turnover?
- 2. Does the support of innovation by firms affect their decision to acquire new patents?
- 3. Does the support of innovation make the firms launch new products?
The questions that were used in the questionnaire were developed to go in line with the research questions. The strengths and weaknesses of the method used were analyzed in depth in chapter 3. We used telephone interviews since it was economical and allowed managing the questionnaires easier.
The case study for this study was firms in Taiwan that were involved in the medical and biotechnology industry.
5.2 Discussion
Based on the data collected and analyzed, it was evident from the regression analysis that:
- 1. Interrelation between firms and buying/selling of patents by firms were the two factors that affected their turnover attributed to technology.
- 2. Buying/selling of patents was the only factor that significantly affected the firms’ decision to acquire new patents.
- 3. Alliance with the universities and interrelationship between firms affected their decisions to launch new products.
Several researches have examined the relationship between innovation and performance. Although the basic finding regarding the impact of innovation on performance is positive, different researches have showed different factors as triggers for bringing better performance to organizations. Dierickx and Cool (1989) suggest that if technology-based firms own more existing knowledge stocks, they do not only exploit more stocks of knowledge but also facilitate their willingness to gain new knowledge. Decarolis and Deeds (1999) agree to this concept and state that firms located in a place with a convergence of many similar firms or with more research institutes and trained labours have better performance than the geographically isolated firms due to the access of the knowledge flows. Moreover, they argue that the higher number of strategic alliances of a firm do not have positive impact on a company’s performance because the quality of alliances and whether knowledge actually flows into a firm or not cannot be guaranteed. This shows that the results from our study are consistent with the existing literature concerning the impact of innovation on the general performance in terms of output, the number of patents acquired, and the ability to launch new products.
5.3 Implications for Management
There are various practical implications derived from the above study. This is because innovation has a positive impact on the production, as well as the ability to produce new products and acquire new patents (Beiske 2002).
This is a challenge to the management of firms to implement policies that encourage innovation in order to improve productivity of the firms and encourage new methods of production. The firms are encouraged to have alliances with institutions of higher learning in their respective location areas.
5.4 Limitations of the study
The research study had several limitations, such as:
- The sample size was very small, and therefore, the study might not reflect the true picture of the situations of the firms all over the world.
- The research might be biased due to the use of the phone interviews that would encourage firms to give the wrong figures of their information (Martin 2002, p. 250).
- The firms that were used in this study only came from the biotechnology industry. Therefore, the study cannot be generalized for all the firms in different industries (Beiske 2002).
5.5 Recommendations for Future Research
The study has left lot of room for research because it is not possible to carry out a perfect research (Cooper et al 2001, p. 213).
More research should be done on the particular methods of innovation that firms use in order to boost the validity of this research study.
Another recommendation is that the study should be carried out in many firms around the world so that the conclusions obtained can be generalized for firms even in other areas of production.
5.6 Conclusion
The study focused on the impact of innovation on firms in terms of their production capacity, the ability to acquire new patents, and to produce new commodities or products. It has been proved that the firms ought to either collaborate with universities and institutions of higher learning or interrelate with other firms for production purposes.
This study has strengthened other studies that were carried out in the same field and could be used to provide practical methods for firm managers in order to improve their productivity and invent new methods of production. This will help improve the current status of production of various firms.